Your cart is currently empty!
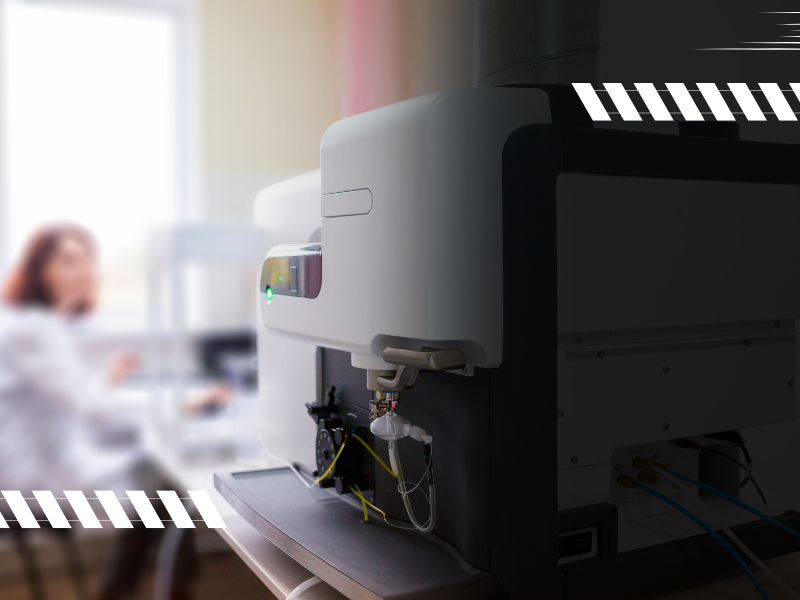
Near-Infrared Spectroscopy (NIR) + AI
Chapter 1: Understanding Near-Infrared Spectroscopy (NIR)
1.1 What is NIR?
NIR spectroscopy is a method that uses the near-infrared region of the electromagnetic spectrum (approximately 700 nm to 2500 nm) to assess the composition of organic materials. The core principle is that when materials are exposed to NIR light, certain wavelengths are absorbed by the molecules in the material, depending on the molecular structure. The amount of light absorbed at specific wavelengths gives insights into the chemical composition of the material.
NIR is particularly well-suited for analyzing organic compounds because it is sensitive to the bonds between hydrogen, carbon, and oxygen. This makes it ideal for analyzing water, proteins, fats, and carbohydrates—substances commonly found in organic materials.
1.2 How AI Enhances NIR
The addition of AI to NIR systems allows for real-time data analysis and the continuous improvement of predictive models. Here’s how AI enhances NIR:
- Pattern Recognition: AI algorithms can detect patterns in NIR data that may not be immediately apparent. This is especially useful when analyzing complex materials with overlapping spectral features.
- Data Calibration and Prediction: AI can build predictive models that continuously improve as more data is gathered. These models can predict outcomes such as composition or quality based on NIR readings, reducing the need for repetitive calibration.
- Real-Time Analysis: AI allows for faster interpretation of NIR data, enabling real-time decision-making in industries such as agriculture and pharmaceuticals.
- Automation and Adaptability: AI-powered NIR systems can be adapted to different applications without needing to manually adjust the system for each use case.

Chapter 2: Key Benefits of NIR with AI
2.1 Speed and Efficiency
NIR is inherently fast as it does not require time-consuming sample preparation. The integration of AI enhances this speed by automating data analysis, reducing the time needed to interpret results. In industries where rapid decision-making is crucial, such as agriculture or pharmaceuticals, this speed allows businesses to optimize production processes, reduce waste, and improve overall efficiency.
2.2 Accuracy and Precision
While traditional NIR systems are accurate, they are often limited by the complexity of the samples being analyzed. AI improves precision by learning from data over time, correcting for variables such as temperature, humidity, and sample variations. AI’s ability to process large datasets and refine predictions means that over time, the system becomes more accurate, even in complex or noisy environments.
2.3 Cost-Effectiveness
The combination of NIR and AI reduces costs in several ways:
- Lower Labor Costs: Automated analysis decreases the need for manual interpretation of data.
- Reduced Material Waste: AI allows for more precise monitoring of processes, reducing material waste in industries like manufacturing and agriculture.
- Lower Infrastructure Costs: Since NIR is non-destructive, the same sample can be used for multiple tests without the need for expensive reagents or destructive sampling.
2.4 Real-Time Monitoring and Control
AI-enhanced NIR systems provide real-time feedback, which is invaluable for process control in industries like food production and pharmaceuticals. By continuously monitoring product quality during production, adjustments can be made on the fly, ensuring consistent output and reducing the risk of defects.
2.5 Environmental Sustainability
The ability to make real-time adjustments to processes also supports sustainability initiatives. For example, in agriculture, farmers can adjust irrigation and fertilization in real-time based on NIR data, reducing the overuse of water and chemicals, and promoting more sustainable farming practices.

Chapter 3: Applications of NIR with AI Across Industries
NIR spectroscopy, when combined with AI, is transforming a wide array of industries. Here are five key examples where NIR with AI is having a significant impact:
3.1 Agriculture: Precision Farming
In modern agriculture, there is increasing demand for methods that can improve yield, reduce input costs, and promote sustainability. NIR with AI is being used to analyze soil and crop health, optimizing the use of fertilizers, water, and pesticides.
- Use Case: Soil Analysis
- NIR technology is used to measure soil nutrient levels, moisture content, and organic matter in real time. The AI component helps predict soil fertility trends based on past data, allowing farmers to apply fertilizers more efficiently, reducing costs and environmental impact.
- Benefit: Farmers can make data-driven decisions about when and how much fertilizer or water to use, improving crop yields while minimizing waste.
- Use Case: Crop Health Monitoring
- NIR can assess the health of crops by analyzing the reflection of NIR light from plant leaves. AI processes these reflections to detect signs of disease, nutrient deficiencies, or stress earlier than traditional visual inspections.
- Benefit: Early detection of issues allows for timely interventions, leading to healthier crops and higher yields.
Example in Practice: Companies like ChrysaLabs use portable NIR devices powered by AI to offer real-time soil analysis. This helps farmers optimize resource usage, leading to more sustainable farming practices.

3.2 Pharmaceuticals: Quality Control and Process Optimization
In the pharmaceutical industry, maintaining consistent product quality is critical, and NIR with AI plays a vital role in ensuring this. AI-powered NIR spectroscopy is used to analyze the chemical composition of drugs and active pharmaceutical ingredients (APIs) during production.
- Use Case: Real-Time Release Testing (RTRT)
- NIR combined with AI allows pharmaceutical companies to perform non-destructive quality testing of drug formulations in real time, ensuring that each batch meets the required chemical composition before it leaves the production line.
- Benefit: This reduces the need for post-production quality checks, speeds up the release of products, and ensures that defective batches are identified early in the process.
- Use Case: Blend Uniformity
- In tablet production, it is essential that the ingredients are uniformly mixed to ensure the correct dosage. AI-enhanced NIR can measure the uniformity of blends in real time, making adjustments as needed during production.
- Benefit: This improves product consistency and reduces the likelihood of costly recalls.
Example in Practice: Companies like Pfizer and GSK have implemented AI-powered NIR systems to streamline their manufacturing processes and enhance quality control, particularly for sensitive products such as vaccines and biologics.

3.3 Food and Beverage: Ensuring Product Quality
The food and beverage industry requires stringent quality control to ensure that products meet safety and nutritional standards. NIR with AI is commonly used for the non-destructive analysis of food composition, moisture levels, and contamination.
- Use Case: Nutrient Content Analysis
- NIR is used to measure the protein, fat, and carbohydrate content of foods such as dairy products, meats, and grains. AI algorithms can learn from NIR spectra to detect subtle changes in nutrient composition over time.
- Benefit: Ensuring consistent quality in terms of nutritional content helps food manufacturers meet regulatory standards and maintain consumer trust.
- Use Case: Moisture Content Monitoring
- Moisture content in foods like cereals, coffee, and powdered products can significantly impact shelf life and product quality. AI-enhanced NIR can monitor moisture levels in real time during processing, ensuring that the final product meets the required specifications.
- Benefit: This reduces spoilage and extends product shelf life, while also preventing product degradation due to moisture fluctuations.
Example in Practice: Companies like Nestlé and Unilever use NIR with AI in their production lines to ensure the consistency of their food products, improving both quality and efficiency.

3.4 Healthcare: Non-Invasive Medical Diagnostics
In healthcare, the development of non-invasive diagnostic tools is revolutionizing patient care. NIR spectroscopy, combined with AI, is emerging as a tool for diagnosing diseases without the need for invasive procedures.
- Use Case: Glucose Monitoring
- For diabetic patients, continuous glucose monitoring is essential. NIR with AI can non-invasively measure glucose levels in the blood through the skin, eliminating the need for finger pricks. AI algorithms process the NIR data to provide accurate glucose readings.
- Benefit: This improves the quality of life for diabetic patients and allows for continuous monitoring, which is crucial for effective disease management.
- Use Case: Tissue Oxygenation Monitoring
- NIR is used to measure oxygen levels in tissues, which can provide insights into conditions such as hypoxia or poor circulation. AI enhances the interpretation of these readings, offering real-time insights for critical care monitoring.
- Benefit: Real-time monitoring allows for quicker responses to changing patient conditions, particularly in surgical or ICU settings.
Example in Practice: Devices like the OxiTone wrist-worn monitor use NIR and AI to non-invasively measure blood oxygen levels, offering real-time health data for patients in critical care settings.

3.5 Environmental Science: Monitoring and Managing Resources
Environmental monitoring often requires the rapid assessment of soil, water, and air quality. NIR combined with AI is being used to track and manage environmental resources more effectively, ensuring sustainability and compliance with environmental regulations.
- Use Case: Water Quality Monitoring
- NIR with AI can assess water quality by analyzing organic and inorganic pollutants. AI models can detect trends in water contamination and predict future risks, helping to prevent environmental disasters.
- Benefit: Continuous monitoring allows for early intervention in case of contamination, protecting ecosystems and public health.
- Use Case: Soil Contamination Detection
- NIR can detect contaminants in soil, such as heavy metals or chemical pollutants, which are often invisible to the naked eye. AI enhances this process by learning from past data to improve the accuracy of contamination detection.
- Benefit: Early detection of soil contamination enables faster remediation efforts, reducing environmental damage and associated costs.
Example in Practice: Organizations like The Nature Conservancy use AI-powered NIR systems to monitor and manage environmental resources more effectively, ensuring sustainable practices in agriculture and conservation.

Conclusion
The combination of Near-Infrared Spectroscopy (NIR) with Artificial Intelligence (AI) offers significant benefits across a wide range of industries. From agriculture and pharmaceuticals to food production and environmental science, the integration of AI enhances NIR’s capabilities, allowing for faster, more accurate, and cost-effective analyses. As industries continue to embrace data-driven decision-making, the role of NIR with AI will only expand, leading to innovations that improve efficiency, product quality, and sustainability.
The examples provided in this report illustrate the transformative impact of NIR with AI, highlighting its versatility and the broad potential for future applications. By enabling real-time monitoring and data analysis, NIR with AI is not only improving operational efficiency but also driving sustainable practices and improving health outcomes across the globe.